Implementing Agentic GenAI in Your Organization: A Strategic Guide for Decision Makers
The Game-Changing Power of Agentic AI In today's rapidly evolving technological landscape, Agentic Generative AI represents a paradigm shift for organizations seeking significant competitive advantage. Unlike traditional AI systems that respond to specific commands, agentic AI systems can autonomously plan, reason, and execute complex tasks with minimal human intervention. For startups and established enterprises alike, effective implementation of these systems can dramatically enhance productivity, drive innovation, and create new revenue streams. However, successful integration requires strategic planning, clear understanding of capabilities, and alignment with business objectives.
#AI#COPILOT#LLMS #AIFUTURE#INNOVATIONINAI #TECHTRENDS #AIACCESSIBILITY#CREATIVEAI #DIGITALTRANSFORMATION#AGENTICAI#GENAI
David Kohav
3/23/20254 min read
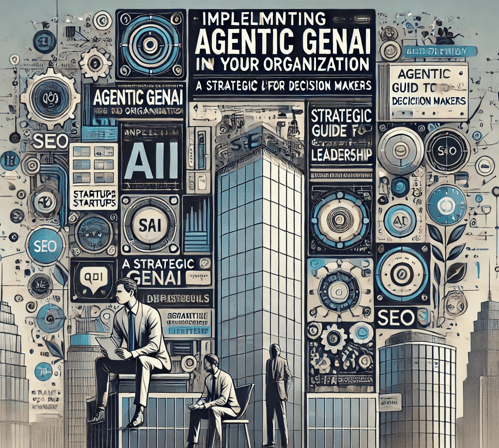
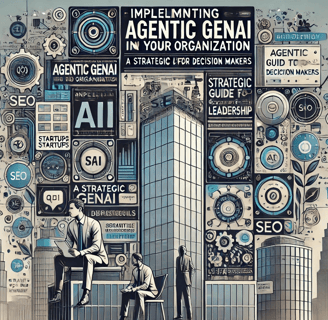
Introduction: The Game-Changing Power of Agentic AI
In today's rapidly evolving technological landscape, Agentic Generative AI represents a paradigm shift for organizations seeking significant competitive advantage. Unlike traditional AI systems that respond to specific commands, agentic AI systems can autonomously plan, reason, and execute complex tasks with minimal human intervention.
For startups and established enterprises alike, effective implementation of these systems can dramatically enhance productivity, drive innovation, and create new revenue streams. However, successful integration requires strategic planning, clear understanding of capabilities, and alignment with business objectives.
What Exactly is Agentic GenAI?
Agentic GenAI combines the creative capabilities of generative AI with autonomous decision-making frameworks. These systems can:
Plan and execute multi-step tasks independently
Learn from interactions to improve performance over time
Coordinate across different tools and data sources
Adapt to changing conditions without requiring reconfiguration
Operate within guardrails defined by organizational policies
Unlike traditional automation, which follows rigid rules, agentic systems can handle ambiguity, make contextual decisions, and leverage creative problem-solving approaches.
Why Decision Makers Should Prioritize Agentic AI Now
The business case for agentic AI implementation is compelling:
Enhanced Operational Efficiency - Reduce time spent on repetitive cognitive tasks by 40-60%
Accelerated Innovation Cycles - Decrease product development time through autonomous prototyping and testing
Improved Customer Experiences - Deploy personalized, 24/7 intelligent service agents
Competitive Differentiation - Create novel capabilities that are difficult for competitors to replicate
Data-Driven Decision Making - Enable real-time synthesis of complex information from multiple sources
Organizations that delay implementation risk falling behind as early adopters establish new industry benchmarks for productivity and innovation.
Key Components of an Enterprise Agentic AI Architecture
A robust agentic AI implementation typically includes these core elements:
1. Foundation Models Layer
At the core of agentic systems are large language models (LLMs) or multimodal foundation models that provide reasoning capabilities. Key considerations include:
Selecting between hosted API solutions vs. on-premises deployment
Evaluating performance vs. cost tradeoffs across model sizes
Implementing effective prompt engineering strategies
Establishing model evaluation frameworks to ensure quality
2. Knowledge Integration Layer
Agentic systems require access to organizational knowledge through:
Retrieval-Augmented Generation (RAG) frameworks
Vector databases for efficient semantic search
Document processing pipelines
Enterprise data connectors with appropriate access controls
3. Tool Integration Framework
To execute tasks, agents need controlled access to:
Internal applications and APIs
Productivity and communication tools
Specialized domain-specific software
Data analysis and visualization capabilities
4. Orchestration and Memory Systems
Sophisticated agents require:
Planning mechanisms for complex task decomposition
Short and long-term memory stores
Meta-cognitive evaluation capabilities
Multi-agent coordination frameworks
5. Security and Governance Layer
Enterprise implementations must prioritize:
Data privacy controls and audit trails
Output validation mechanisms
Alignment with regulatory requirements
Prompt injection and data leakage prevention
Implementation Roadmap: A Phased Approach
Successful agentic AI integration follows a strategic progression:
Phase 1: Controlled Pilots (2-3 months)
Begin with narrowly-defined use cases that offer measurable ROI:
Document analysis and summarization across legal, financial, or technical domains
Customer support augmentation with human oversight
Internal knowledge base enhancement and accessibility
Data analysis and reporting automation for specific departments
Key success factors include:
Setting clear success metrics aligned with business objectives
Establishing feedback loops with end users
Documenting challenges and lessons learned
Building technical capability within your team
Phase 2: Expanded Deployment (3-6 months)
Based on pilot results, expand to more complex use cases:
Process automation across departmental boundaries
Decision support systems for mid-level managers
Product development acceleration through automated testing and feedback
Customer journey enhancement with personalized interactions
Focus areas should include:
Scaling infrastructure based on usage patterns
Developing robust evaluation frameworks
Establishing governance processes
Creating training programs for users and administrators
Phase 3: Enterprise Integration (6+ months)
Full integration involves:
Workflow reimagination around agentic capabilities
Strategic initiatives powered by autonomous systems
Cross-functional agent ecosystems that share knowledge
New product and service offerings enabled by AI capabilities
Long-term success requires:
Continuous improvement mechanisms
ROI measurement and optimization
Ethical use policies and compliance frameworks
Strategic roadmapping for emergent capabilities
Common Implementation Challenges and Solutions
Challenge 1: Integration Complexity
Solution: Implement a modular architecture with well-defined APIs between components, allowing incremental deployment and updates.
Challenge 2: Data Quality Issues
Solution: Develop robust data preprocessing pipelines and implement feedback mechanisms to identify and correct inaccuracies.
Challenge 3: User Adoption Resistance
Solution: Focus on augmentation rather than replacement, with transparent explanation of capabilities and limitations.
Challenge 4: Security and Compliance Concerns
Solution: Implement comprehensive logging, access controls, and regular security audits while maintaining clear data governance policies.
Challenge 5: Cost Management
Solution: Develop usage monitoring tools, implement caching strategies, and optimize prompt designs to reduce token consumption.
Case Studies: Game-Changing Implementations
Financial Services: JPMorgan Chase
JPMorgan Chase, one of the world's largest financial services companies, has implemented AI agents across its systems. The firm leverages AI for fraud detection, automation of financial processes, and enhancing customer experience through intelligent chatbots that provide personalized financial information and responses to customers
Service and Support: ServiceNow
ServiceNow reported savings of more than $350 million in the past year through the use of autonomous AI agents across various domains including customer service, IT support, and human resources.
Manufacturing: Protolabs
Protolabs, a global leader in on-demand manufacturing, has integrated advanced technologies and AI systems into its production processes. The company offers various services such as 3D printing, CNC machining, and injection molding, using intelligent systems to optimize manufacturing processes and improve efficiency. The implementation of AI enables Protolabs to quickly respond to changing market demands and provide customized manufacturing solutions to its customers, significantly reducing delivery times and increasing flexibility in manufacturing processes.
The Future: Emergent Capabilities and Competitive Advantage
Organizations that successfully implement agentic AI today are positioned to benefit from rapid advancements in:
Multi-agent collaboration systems that simulate organizational structures
Embodied AI integration with robotics and IoT systems
Specialized domain adaptation of foundation models
Autonomous strategy development and scenario planning
These capabilities will increasingly define competitive differentiation across industries.
Conclusion: Strategic Imperative for Decision Makers
Implementing agentic GenAI is no longer optional for organizations seeking to remain competitive. The question is not whether to adopt these technologies, but how quickly and effectively they can be integrated into core business operations.
Decision makers should:
Identify high-value use cases specific to their organization
Allocate resources for strategic implementation
Develop internal expertise through training and hiring
Establish governance frameworks that enable innovation while managing risk
Create feedback mechanisms for continuous improvement
The organizations that act decisively now will establish lasting competitive advantages as agentic AI becomes the foundation of next-generation enterprise systems.
Ready to Transform Your Organization with Agentic GenAI?
Contact our team of implementation specialists to develop a customized roadmap for your specific business needs. Schedule a consultation today to begin your AI transformation journey.
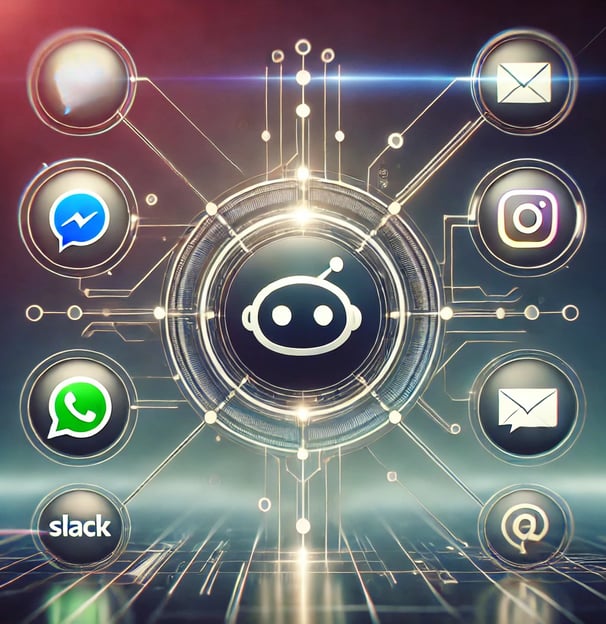
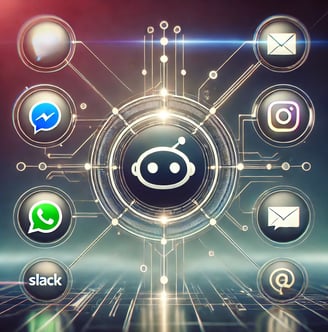
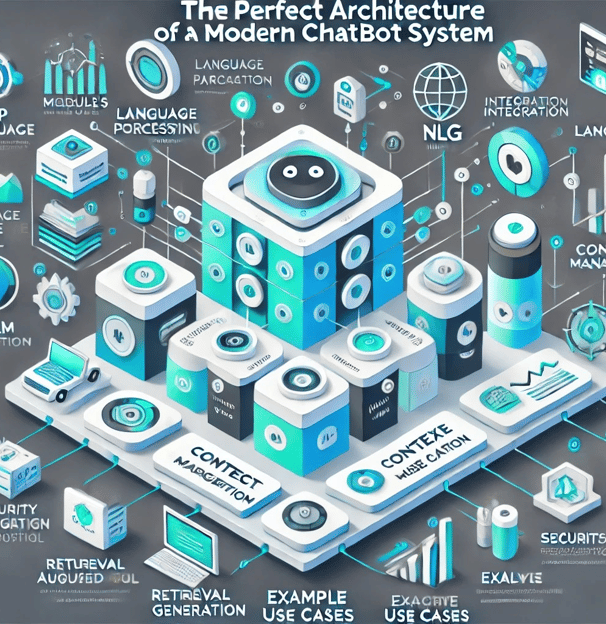
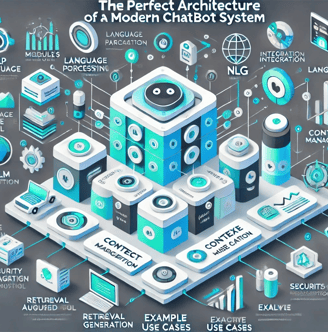